Making the web maps smarter
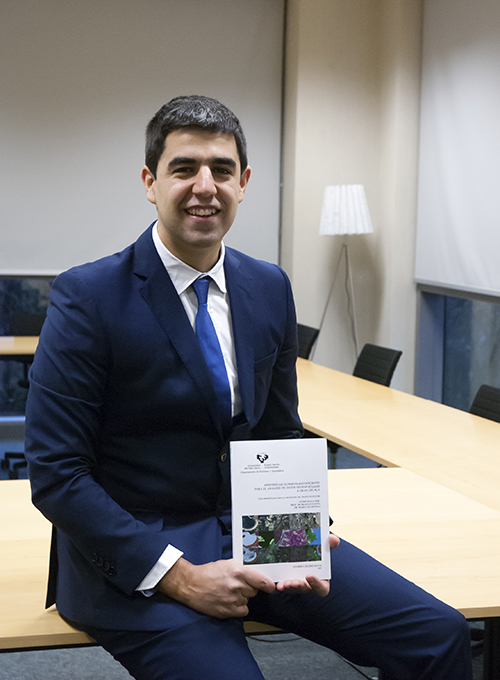
21.01.2016
Javier Lozano, researcher in the Digital Media department of Vicomtech-IK4 has recently presented his thesis “Large scale geospatial data analysis through efficient supervised machine learning”.
This scientific work developed by Javier Lozano in Vicomtech-IK4 and directed by Dr. Ekaitz Zulueta from Universidad del País Vasco and Dr. Marco Quartulli from Vicomtech-IK4 is an important step towards the web-based automatic learning techniques integration in map and tele detection data servers.
This system answers to the search queries made by users with a thematic map, on a map generated by high resolution images taken by an aerial camera.
This way, different user profiles, even people without tele detection knowledge, could generate updated maps, for example related to the following issues: growth of urban areas, environmental degradation and desertification processes.
Different elements are combined in the thesis, such as automatic learning techniques, map servers and system development which allow the interaction of the user with the system.
During the development of the doctoral thesis an approach of the developed platform to the big data environments has been made, allowing to validate its feasibility and behaviour to face continental and global scope problems.
The objective of integrating the automatic learning in web servers has been achieved for the first time through this work
Technical Abstract
Large scale geospatial data analysis through efficient supervised machine learning
The present thesis aims to test the viability of the integration of machine learning capabilities into web map servers. The validation of this hypothesis has been carried out by the development of a pre-operational prototype. The developed prototype is a platform for thematic mapping by supervised learning from very high resolution remote sensing imagery data through a web platform. This contribution overcomes the current state of art, characterized by the separation of the two areas, which requires a continuous involvement of remote sensing experts in thematic mapping intensive tasks: labour intensive tasks are supplemented by the integration of the scalability capabilities from machine learning engines and web map servers. With this hypothesis the application field referred to the semi-automatic creation of large scale thematic maps can open up different fields, from agriculture to the environmental monitoring field, to expert users of these applications domains with limited specific knowledge of remote sensing techniques. Semantic tagging algorithms based on supervised classification methods can be exploited for thematic map creation from raster data based on user needs. This requires the integration of machine learning capabilities within web map servers, along with a simple interface that enables navigation and the monitoring of geospatial learning. The adaptive nature of this learning, along with its integration into a web server, requires a classification algorithm characterized by efficient management and processing of data in time scales compatible with traditional web browsing. At the same time, the volume of data managed by remote sensing applications motivates the transfer of the developed methodology to cloud environments under the Big Data paradigm.