The challenges of connected, cooperative and automated mobility (CCAM)
By Dr. Oihana Otaegui, Transport and Security Director, Vicomtech.
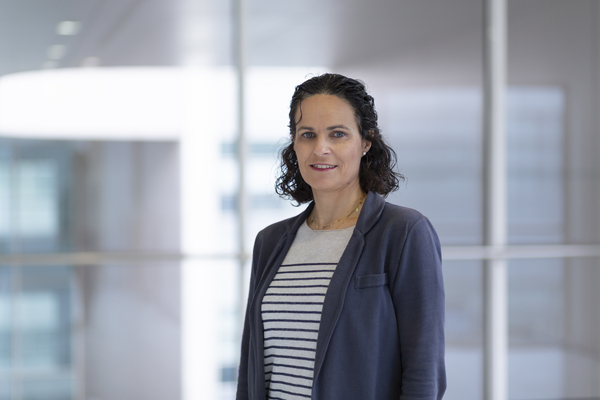
22.02.2022
Mobility is facing one of the greatest challenges in its history "towards zero" which considers that mobility must achieve the goals of 0 deaths, 0 emissions and 0 traffic jams, which entails having to act on all fronts and elements in mobility, vehicles, propulsion, sensors, infrastructure and traffic management.
Regarding 0 emissions we can say that greenhouse gas emissions related to energy production are the main cause of climate change. By 2050, around 70% of the world's population will live in cities and overall growth could add 2.5 billion people to urban areas. Urbanization generates enormous consequences in terms of the mobility of people and goods, and cities will become critically dependent on their transport systems. Failures in transport systems lead to delays, increased costs, frustration, increased pollution, and poor health. The World Health Organization estimates that air pollution causes 4.2 million premature deaths each year worldwide, of which about 400,000 occur in the EU according to the EEA (2019). Awareness of air pollution (and exposure to small PM10 and PM2.5 particles) as a major health issue is growing, and transportation is a significant contributor to such emissions. Transport accounts for up to 50% of PM emissions in OECD countries. With population growth, urbanization and environmental needs, transport regulations regarding greenhouse gas emissions become more stringent and cities need to adapt infrastructure to change behavior and improve life. Efficient traffic and network management solutions are needed, but the implementation of these systems depends directly on the state of the technologies that help fully optimize traffic, such as assisted or automated vehicles, telecommunications, and infrastructure sensorization.
Regarding the automation of driving to optimize the driving task and avoid accidents, it must be said that driving is a very complex task, which requires the cooperation of several cognitive and psychomotor functions at the same time. Accidents can be the result of many different factors, which can be classified into three categories: the road, the vehicle, and the driver. Despite all the other factors on the road, human error remains the deadliest factor (90% of traffic accidents are caused by human error). According to the World Health Organization, more than 1.24 million people worldwide died in 2010 from road traffic injuries.
We are in a time of profound changes in the way we get around thanks to the arrival of electric vehicles with longer-range batteries and autonomous driving technology that is in full development. Autonomous vehicles will favor the large-scale penetration of electric mobility and thus increase its advantages in terms of not only environmental sustainability. On the one hand, automation itself can improve energy efficiency, since it reduces acceleration and braking and is able to maintain a better constant flow of vehicles in the infrastructure. On the other hand, these vehicles can be linked to other innovations in the mobility sector such as shared cars or mobility-as-a-service (mobility as a service or MaaS), which provide integrated transport services through subscription.
Embedded systems and vehicles with autonomous functionalities
The introduction of innovative Connected, Cooperative and Automated Mobility (CCAM) has the potential to make a strong contribution to improving transport in Europe. It can lead to healthier, safer, more accessible, sustainable, cost-effective, and demand-responsive transport everywhere and for everyone. The introduction of connected automated vehicles (CAV) requires reliable and affordable on-board decision-making and perception systems.
The last decade has shown that AI based on deep learning is indispensable in the development of these future perception and decision-making technologies. Still, it has some key shortcomings that are related to its limited generalizability and lack of traceability (failure cases) that prevent its certifiable implementation, largely due to the lack of sufficient monitoring data. high quality training. What is required for a successful AI implementation is a combination of learning-driven (data) and model-driven AI. This will allow deep learning capabilities to be integrated into model-based methods for safety, transparency, and traceability, thus achieving safe and reliable interaction between vehicles, infrastructure, and road users.
That is why the next-generation vehicle sensor suite will consist of a combination of existing sensors that meets cost, functionality, and accuracy requirements (accurate, more reliable, and more resistant to adverse conditions). These sensors such as cameras, lidars and radars will be complemented by the design of robust AI-based algorithms that will generate greater situational awareness to enable safe decision-making.
It should be noted that for the research and development of AI-based perception and decision systems, massive amounts of raw (real recordings) and processed data are required to facilitate the training and validation process of these systems. This data management is being a big bottleneck today.
At first glance, autonomous driving (SAE-L3 and above) could be seen as a simple continuation of the development of advanced driver assistance systems (SAE-L2), which help the driver with specific driving actions, such as changing lanes, keep the front distance and emergency braking. However, this step forward implies a great challenge, considering the performance and reliability requirements demanded of the systems. Drivers (humans) statistically drive 7.5 million km without any accidents. The inclusion of SAE-L2 ADAS systems reduces the probability of an accident by a factor of ten. Therefore, autonomous vehicles with automation level SAE-L3 or higher face the challenge of avoiding or controlling any critical situation within a statistical distance of at least 75 million km between accidents, in order to achieve performance similar to of an SAE-L2 (assisted driving).
This distance necessarily includes an extremely variable range of roads and driving situations, which a human driver can handle without any problem, but which can be challenging for automated systems. Therefore, test procedures and methodologies for SAE-L3 remain a key unresolved challenge for validation of highly automated driving. The starting point is the current SAE-L2 test approaches, which basically define all possible scenarios, create real recordings with test vehicles of such situations, or generate simulations and allow evaluation of the performance of the system under test. In order to provide answers to such questions, the industry must address the following technological issues:
- Appropriate test environment and standardization of methodologies.
- Smart raw data management
- Provide a framework to enhance and manage scene description data
- High-performance computing and application scalability
- Enable interoperability and complex multi-domain simulation
Finally, it is worth mentioning that management at the macroscopic level for both individual vehicles and fleet management will play an important role in the organization of electric mobility. Systems for recharging management optimization and battery autonomy extension will play a key role in promoting the deployment of electric mobility. We must not forget the importance of efficient route management and charging planning based on the extraction of driving profiles, routes, and battery characterization to reduce the driver's "range anxiety" by providing individualized information. In addition, the development and implementation of recommendation engines in real time that offer safe alternatives to the driver based on consumption during the route, since this can be easily altered by external factors (weather, topography of the terrain, delays, or changes in routes) or by factors inherent to the driver himself (driving profile, comfort level).